GenAI Weekly News Update 2024-11-18
News Update
Research Update
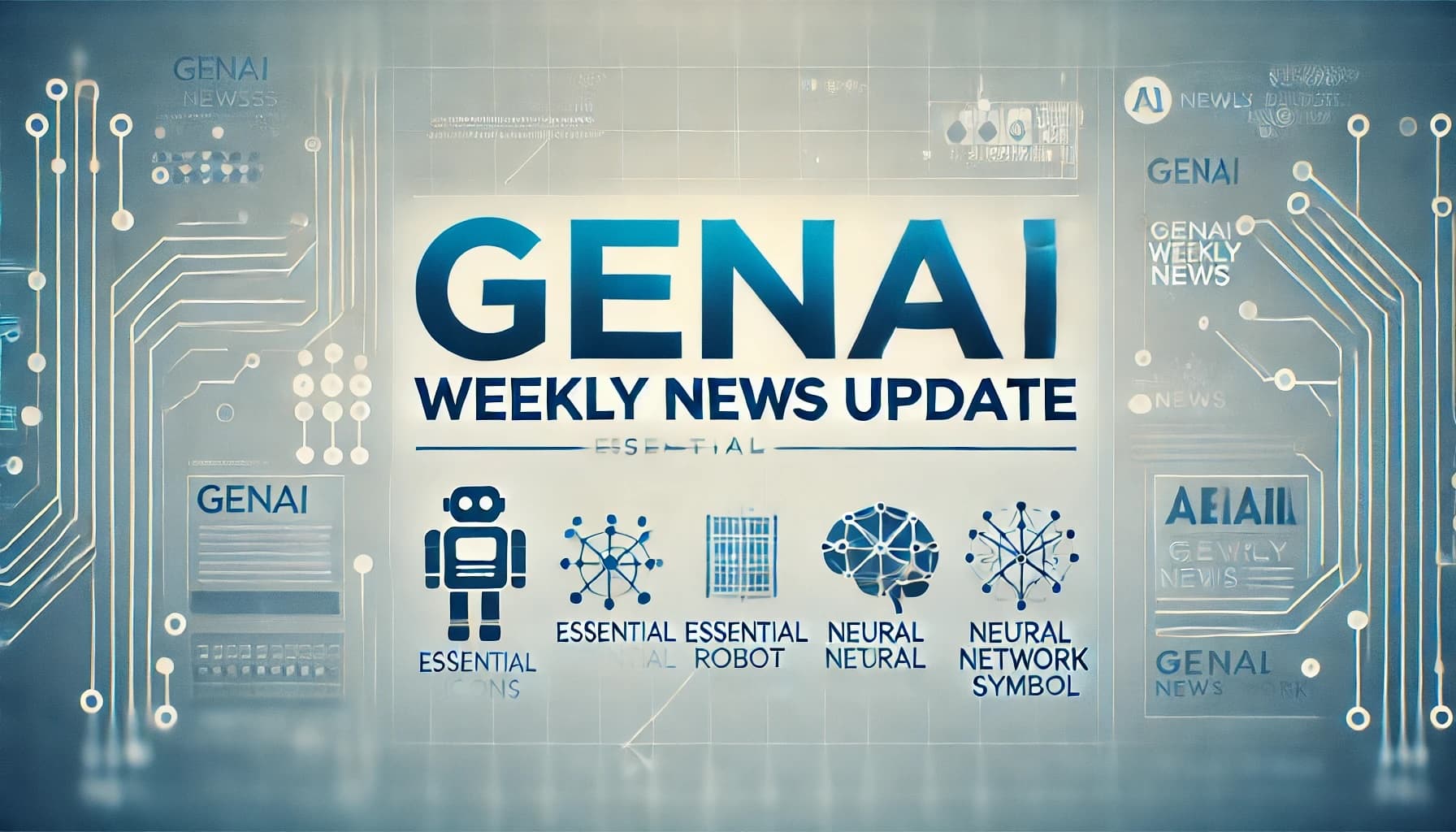
The Allen Institute for AI introduced Tülu 3, an open-source model promoting transparency by providing access to all data and methodologies, while Mistral AI enhanced its generative assistant, Le Chat, with real-time web search, ideation tools, and multimodal capabilities. Black Forest Labs launched FLUX.1 Tools for advanced image editing, offering unparalleled precision in recreating and modifying images. Google and OpenAI escalated their AI rivalry, with Google’s Gemini model outperforming GPT-4o and OpenAI exploring browser integration for ChatGPT. Anthropic and Amazon deepened their partnership with a $4 billion investment, focusing on AI hardware and integrations like Claude with Amazon Bedrock. Finally, new research emphasized adding statistical rigor to language model evaluations and introduced Marco-o1, a reasoning model leveraging advanced techniques for improved problem-solving.
Model Update
Ai2 Unveil the Full Transparency Tülu 3 Model
The Allen Institute for AI (Ai2) has unveiled Tülu 3, a suite of open-source, post-trained language models designed to bridge the performance gap between open and proprietary AI systems. Unlike many closed models that withhold training data and methodologies, Tülu 3 offers full transparency by providing access to its data, training recipes, code, infrastructure, and evaluation frameworks.
Tülu 3's development involved creating new datasets and training procedures, including methods for training on verifiable problems using reinforcement learning and generating high-performance preference data from the model's own outputs. This comprehensive approach aims to enhance specialized capabilities without compromising general performance.
By releasing Tülu 3, Ai2 seeks to empower researchers, developers, and AI practitioners to post-train open-source models to a quality comparable to leading closed models, fostering innovation and transparency in AI development.
Mistral AI announced significant enhancements to Le Chat
Mistral AI has announced significant enhancements to its free generative AI assistant, Le Chat, introducing features aimed at boosting productivity and creativity.
- Web Search with Citations: Le Chat now integrates real-time internet search capabilities, providing users with up-to-date information accompanied by proper citations.
- Canvas for Ideation: A new interface allows users to collaborate with Mistral's language models on shared outputs, facilitating the creation and editing of documents, presentations, code, and more.
- Advanced Document and Image Understanding: Powered by the new multimodal Pixtral Large model, Le Chat can process and analyze complex PDFs and images, extracting insights from various elements such as graphs, tables, and equations.
- Image Generation: Through a partnership with Black Forest Labs, Le Chat now offers high-quality image generation capabilities, enabling users to create visuals directly within the platform.
These enhancements position Le Chat as a comprehensive tool for professionals and creatives, offering a unified platform that combines advanced AI functionalities with user-friendly interfaces.
Black Forest Labs Launches FLUX.1 Tools for Advanced Image Generation and Editing
Black Forest Labs has unveiled FLUX.1 Tools, a cutting-edge collection of image generation and editing models that provide users with enhanced control and flexibility. These tools, built on the FLUX.1 text-to-image base model, enable seamless modification and recreation of both real and AI-generated images.
Key features include:
- FLUX.1 Fill: Allows detailed inpainting and outpainting using text prompts and binary masks for precise editing.
- FLUX.1 Depth: Utilizes depth maps for structural guidance, blending input images with creative text prompts.
- FLUX.1 Canny: Integrates canny edge detection to maintain structural integrity during image transformation.
- FLUX.1 Redux: Offers advanced tools for blending, remixing, and recreating images based on user inputs.
These tools are part of the FLUX.1 [dev] series and are available as open-access models. They are also integrated into the BFL API and supported by partners such as fal.ai, Replicate, Together.ai, and Freepik.
The launch reinforces Black Forest Labs’ commitment to advancing open-weight models for researchers while delivering premium capabilities for professional applications.
Google and OpenAI Intensify AI Competition
In the past week, the competition between Google and OpenAI in the development of large language models (LLMs) has intensified, with both companies making significant advancements.
Google's Gemini Model Achievements
Google's DeepMind introduced the Gemini-Exp-1114 model, which has demonstrated performance on par with OpenAI's latest GPT-4o and surpasses the o1-preview reasoning model. This positions Gemini-Exp-1114 at the forefront of current LLM capabilities.
OpenAI's Strategic Moves
In response, OpenAI is reportedly exploring the development of a web browser integrated with its ChatGPT chatbot. This initiative aims to challenge Google's dominance in the search and browser markets, potentially reshaping user interactions with AI-driven search tools.
Industry Implications
These developments underscore the escalating competition between Google and OpenAI to lead in AI innovation. The introduction of advanced models like Gemini-Exp-1114 and OpenAI's potential entry into the browser market highlight the dynamic nature of the AI landscape, with both companies striving to enhance user experiences and expand their technological influence.
AI Company News
Anthropic and Amazon Deepen Partnership with $4 Billion Investment and Trainium Collaboration
Anthropic has expanded its collaboration with Amazon Web Services (AWS) through an additional $4 billion investment from Amazon, bringing its total investment in Anthropic to $8 billion. This partnership designates AWS as Anthropic's primary cloud and training partner.
A key aspect of this collaboration involves Anthropic working closely with AWS's Annapurna Labs to develop and optimize future generations of Trainium accelerators. This joint effort aims to enhance machine learning hardware capabilities, enabling Anthropic to train advanced AI models more efficiently.
Additionally, Anthropic's AI assistant, Claude, is now integrated into Amazon Bedrock, providing tens of thousands of companies with scalable AI solutions. Organizations such as Pfizer, Intuit, Perplexity, and the European Parliament are utilizing Claude through Amazon Bedrock to improve research, customer service, and data analysis processes.
This partnership underscores a commitment to advancing AI research and development, offering secure, customizable AI solutions to a broad range of industries.
AI Research Update
Adding Error Bars to Evals: A Statistical Approach to Language Model Evaluations
Research Background
- Language Model Evaluations: The paper discusses the common practice of evaluating language models using metrics where higher scores are deemed better, often without assessing statistical significance. Traditional evaluations lack confidence intervals, which are crucial for understanding the reliability of results.
- Need for Statistical Rigor: The authors aim to introduce a more rigorous statistical framework for evaluating language models, allowing researchers to quantify the precision of their evaluations and test hypotheses effectively.
Contribution
- Analytic Framework Development: The paper presents a comprehensive framework for computing confidence intervals and reporting evaluation results, emphasizing the importance of statistical significance in model comparisons.
- Key Recommendations:
- Standard Errors: Compute standard errors of the mean using the Central Limit Theorem (C.L.T.) and clustered standard errors for related groups of questions.
- Variance Reduction Techniques:
- Resampling answers to reduce variance.
- Analyzing next-token probabilities to eliminate conditional variance.
- Conducting statistical inference on question-level paired differences rather than population-level summaries.
- Formulas Introduced:
- Standard error of the mean: \mathrm{SE}_{\rm C.L.T.} = \sqrt{\frac{\mathrm{Var}(s)}{n}}
- Confidence interval: \mathrm{CI}{95%} = \bar{s} \pm 1.96 \times \mathrm{SE}{\rm C.L.T.}
- Cluster-adjusted standard error: \mathrm{SE}{\rm clustered} = \left(\mathrm{SE}{\rm C.L.T.}^{2} + \frac{1}{n^{2}} \sum_{c} \sum_{i} \sum_{j \neq i} (s_{i,c} - \bar{s})(s_{j,c} - \bar{s})\right)^{1/2}.
Conclusion and Analysis
- Statistical Significance in Model Comparisons: The paper emphasizes that naive comparisons of model scores can be misleading. By using paired analysis, researchers can achieve a more accurate understanding of model performance, as it accounts for correlations in question difficulty.
- Power Analysis: The authors provide a sample-size formula to determine the number of questions needed to detect a specified effect size with a given power level. This is crucial for designing effective evaluations.
- Final Recommendations: The authors advocate for the inclusion of standard errors in reporting eval scores and suggest that researchers treat model evaluations as experiments rather than mere competitions for the highest scores.
Marco-o1: Towards Open Reasoning Models for Open-Ended Solutions
Research Background
- Motivation: The paper builds on the success of OpenAI's o1 model, which is recognized for its exceptional reasoning capabilities. The authors aim to enhance reasoning abilities in large language models (LLMs) to address complex real-world challenges.
- Existing Techniques: The research incorporates advanced techniques such as Chain-of-Thought (CoT) fine-tuning and Monte Carlo Tree Search (MCTS) to improve reasoning power.
Contribution
- Model Development: Introduction of the Marco-o1 model, which utilizes:
- Fine-Tuning with CoT Data: Full-parameter fine-tuning on the base model using open-source CoT datasets and synthetic data.
- Solution Space Expansion via MCTS: Integration of MCTS to guide the model's search for optimal solutions based on output confidence.
- Reasoning Action Strategy: Implementation of novel strategies that include varying action granularities and a reflection mechanism to enhance problem-solving capabilities.
- Datasets Utilized:
- Open-O1 CoT Dataset (Filtered): Refined dataset for structured reasoning patterns.
- Marco-o1 CoT Dataset (Synthetic): Generated using MCTS to formulate complex reasoning pathways.
- Marco Instruction Dataset: Ensures robust instruction-following capabilities.
- Performance Metrics:
- Achieved accuracy improvements of +6.17% on the MGSM (English) dataset and +5.60% on the MGSM (Chinese) dataset.
- Demonstrated superior translation capabilities, particularly in colloquial expressions.
Conclusion and Analysis
- Effectiveness of MCTS: The integration of MCTS significantly expands the solution space, allowing for exploration of various reasoning paths. The model's performance varies with different action granularities, indicating that finer granularity can enhance problem-solving.
- Reflection Mechanism: The addition of a self-reflection prompt improves the model's accuracy on challenging problems, allowing it to self-correct and refine its reasoning.
- Future Work: Plans to refine the reward signal for MCTS through Outcome Reward Modeling (ORM) and Process Reward Modeling (PRM) to reduce randomness and improve performance further.